Digital soil mapping with regression-Kriging and data from remote sensing
DOI:
https://doi.org/10.28940/terra.v41i0.1617Keywords:
machine learning, pedometrics, scorpanAbstract
The use of digital soil mapping allows obtaining quantitative information on soil distribution and functioning to provide alternatives for their management. The objective of this research is to perform the spatial prediction of soil properties through regression-kriging and serve as a basis for taking the necessary measures for a sustainable use of the resource. The study area is located in the wetland Cienaga de Chapala portion of Michoacán, Mexico. Soil sampling was systematic. In the laboratory, electrical conductivity, organic carbon, soluble cations calcium, potassium, magnesium, sodium (Ca, K, Mg, Na), total nitrogen and pH were determined for each soil sample. The dependent variables were total nitrogen, electrical conductivity, organic carbon and pH. The independent variables are the Landsat 8 satellite bands. Regression-kriging was applied for spatial prediction. The experimental semivariogram fit with theoretical models was consistent for the four soil properties, because it was a systematic sampling design and the sample size was 297 points. The root mean square error of the electrical conductivity spatial prediction was 4 because it showed a coefficient of variation of 355. Soils are considered having from low to very low total nitrogen content (<0.15%). At least 30% of the soils have salinity problems because their electrical conductivity is greater than 1.1 dS m-1. Organic carbon contents are considered medium to high (>0.6%). The pH of the soils is moderately alkaline.
Downloads
Publication Facts
Reviewer profiles N/A
Author statements
- Academic society
- Terra Latinoamericana
- Publisher
- Mexican Society of Soil Science, C.A.
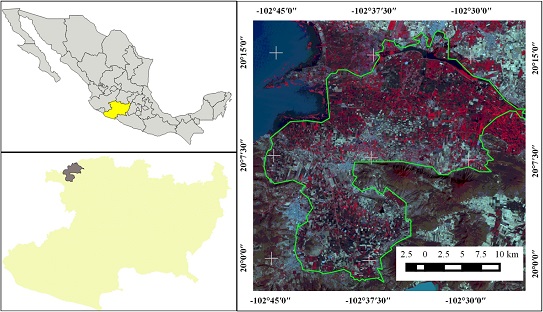